This is a guest post written by Axfood AB. In this post, we share how Axfood, a large Swedish food retailer, improved operations and scalability of their existing artificial intelligence (AI) and machine learning (ML) operations by prototyping in close collaboration with AWS experts and using Amazon SageMaker. Axfood is Sweden’s second largest food retailer, with over 13,000 employees and more than 300 stores. Axfood has a structure with multiple decentralized data science teams with different areas of responsibility. Together with a central data platform team, the data science teams bring innovation and digital transformation through AI and ML solutions to the organization. Axfood has been using Amazon SageMaker to cultivate their data using ML and has had models in production for many years. Lately, the level of sophistication and the sheer number of models in production is increasing exponentially. However, even though the pace of innovation is high, the different teams had developed their own ways of working and were in search of a new MLOps best practice. Our challenge To stay competitive in terms of cloud services and AI/ML, Axfood chose to partner with AWS and has been collaborating with them for many years. During one of our recurring brainstorming sessions with AWS, we were discussing how to best collaborate across teams to increase the pace of innovation and efficiency of data science and ML practitioners. We decided to put in a joint effort to build a prototype on a best practice for MLOps. The aim of the prototype was to build a model template for all data science teams to build scalable and efficient ML models—the foundation to a new generation of AI and ML platforms for Axfood. The template should bridge and combine best practices from AWS ML experts and company-specific best practice models—the best of both worlds. We decided to build a prototype from one of the currently most developed ML models within Axfood: forecasting sales in stores. More specifically, the forecast for fruits and vegetables of upcoming campaigns for food retail stores. Accurate daily forecasting supports the ordering process for the stores, increasing sustainability by minimizing food waste as a result of optimizing sales by accurately predicting the needed in-store stock levels. This was the perfect place to start for our prototype—not only would Axfood gain a new AI/ML platform, but we would also get a chance to benchmark our ML capabilities and learn from leading AWS experts. Our solution: A new ML template on Amazon SageMaker Studio Building a full ML pipeline that is designed for an actual business case can be challenging. In this case, we are developing a forecasting model, so there are two main steps to complete: Train the model to make predictions using historical data. Apply the trained model to make predictions of future events. In Axfood’s case, a well-functioning pipeline for this purpose was already set up using SageMaker notebooks and orchestrated by the third-party workflow management platform Airflow. However, there are many clear benefits of modernizing our ML platform and moving to Amazon SageMaker Studio and Amazon SageMaker Pipelines. Moving to SageMaker Studio provides many predefined out-of-the-box features: Monitoring model and data quality as well as model explainability Built-in integrated development environment (IDE) tools such as debugging Cost/performance monitoring Model acceptance framework Model registry However, the most important incentive for Axfood is the ability to create custom project templates using Amazon SageMaker Projects to be used as a blueprint for all data science teams and ML practitioners. The Axfood team already had a robust and mature level of ML modeling, so the main focus was on building the new architecture. Solution overview Axfood’s proposed new ML framework is structured around two main pipelines: the model build pipeline and the batch inference pipeline: These pipelines are versioned within two separate Git repositories: one build repository and one deploy (inference) repository. Together, they form a robust pipeline for forecasting fruits and vegetables. The pipelines are packaged into a custom project template using SageMaker Projects in integration with a third-party Git repository (Bitbucket) and Bitbucket pipelines for continuous integration and continuous deployment (CI/CD) components. The SageMaker project template includes seed code corresponding to each step of the build and deploy pipelines (we discuss these steps in more detail later in this post) as well as the pipeline definition—the recipe for how the steps should be run. Automation of building new projects based on the template is streamlined through AWS Service Catalog, where a portfolio is created, serving as an abstraction for multiple products. Each product translates into an AWS CloudFormation template, which is deployed when a data scientist creates a new SageMaker project with our MLOps blueprint as the foundation. This activates an AWS Lambda function that creates a Bitbucket project with two repositories—model build and model deploy—containing the seed code. The following diagram illustrates the solution architecture. Workflow A depicts the intricate flow between the two model pipelines—build and inference. Workflow B shows the flow to create a new ML project. Model build pipeline The model build pipeline orchestrates the model’s lifecycle, beginning from preprocessing, moving through training, and culminating in being registered in the model registry: Preprocessing – Here, the SageMaker ScriptProcessor class is employed for feature engineering, resulting in the dataset the model will be trained on. Training and batch transform – Custom training and inference containers from SageMaker are harnessed to train the model on historical data and create predictions on the evaluation data using a SageMaker Estimator and Transformer for the respective tasks. Evaluation – The trained model undergoes evaluation by comparing the generated predictions on the evaluation data to the ground truth using ScriptProcessor. Baseline jobs – The pipeline creates baselines based on statistics in the input data. These are essential for monitoring data and model quality, as well as feature attributions. Model registry – The trained model is registered for future use. The model will be approved by designated data scientists to deploy the model for use in production. For production environments, data ingestion and trigger mechanisms are managed via a primary Airflow orchestration. Meanwhile, during development, the pipeline is activated each time a new commit is introduced to the model build Bitbucket repository. The following figure visualizes the model build pipeline. Batch inference pipeline The batch inference pipeline handles the inference phase, which consists of the following steps: Preprocessing – Data is preprocessed using ScriptProcessor. Batch transform – The model uses the custom inference container with a SageMaker Transformer and generates predictions given the input preprocessed data. The model used is the latest approved trained model in the model registry. Postprocessing – The predictions undergo a series of postprocessing steps using ScriptProcessor. Monitoring – Continuous surveillance completes checks for drifts related to data quality, model quality, and feature attribution. If discrepancies arise, a business logic within the postprocessing script assesses whether retraining the model is necessary. The pipeline is scheduled to run at regular intervals. The following diagram illustrates the batch inference pipeline. Workflow A corresponds to preprocessing, data quality and feature attribution drift checks, inference, and postprocessing. Workflow B corresponds to model quality drift checks. These pipelines are divided because the model quality drift check will only run if new ground truth data is available. SageMaker Model Monitor With Amazon SageMaker Model Monitor integrated, the pipelines benefit from real-time monitoring on the following: Data quality – Monitors any drift or inconsistencies in data Model quality – Watches for any fluctuations in model performance Feature attribution – Checks for drift in feature attributions Monitoring model quality requires access to ground truth data. Although obtaining ground truth can be challenging at times, using data or feature attribution drift monitoring serves as a competent proxy to model quality. Specifically, in the case of data quality drift, the system watches out for the following: Concept drift – This pertains to changes in the correlation between input and output, requiring ground truth Covariate shift – Here, the emphasis is on alterations in the distribution of independent input variables SageMaker Model Monitor’s data drift functionality meticulously captures and scrutinizes the input data, deploying rules and statistical checks. Alerts are raised whenever anomalies are detected. In parallel to using data quality drift checks as a proxy for monitoring model degradation, the system also monitors feature attribution drift using the normalized discounted cumulative gain (NDCG) score. This score is sensitive to both changes in feature attribution ranking order as…
How Axfood enables accelerated machine learning throughout the organization using Amazon SageMaker
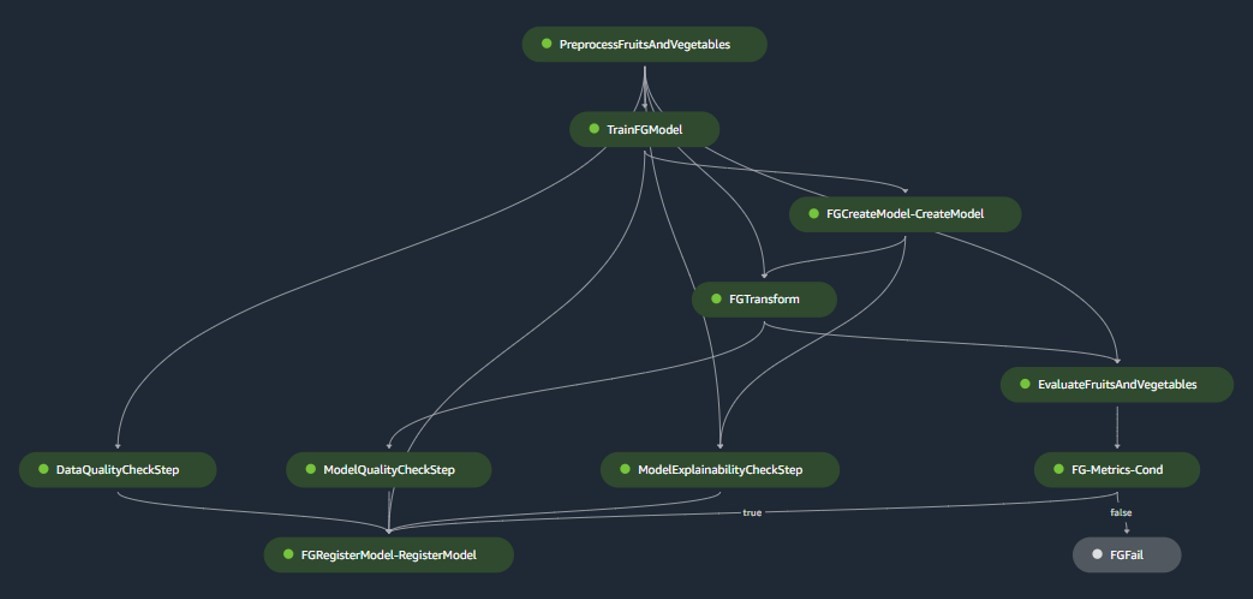
Sign Up for Our Newsletters
Get notified of the best deals on our WordPress themes.