IntroductionWhether you’re a fresher or an experienced professional in the Data industry, did you know that ML models can experience up to a 20% performance drop in their first year? Monitoring these models is crucial, yet it poses challenges such as data changes, concept alterations, and data quality issues. ML Monitoring aids in early detection of model performance dips, data quality issues, and drift problems as new data streams in. This prevents failures in the ML pipeline and alerts the team to resolve the issue. Evidently.ai, a powerful open-source tool, simplifies ML Monitoring by providing pre-built reports and test suites to track data quality, data drift, and model performance. In this beginner’s guide to ML Monitoring with Evidently.ai, you’ll learn effective methods to monitor ML models in production, including monitoring setup, metrics, integrating Evidently.ai into ML lifecycles and workflows, and more.Learning Objectives Apply statistical tests to detect data quality issues like missing values, outliers, and data drift.Track model performance over time by monitoring metrics like accuracy, precision, and recall using Evidently’s predefined reports and test suites.Create a monitoring dashboard with plots like target drift, accuracy trend, and data quality checks using Evidently’s UI and visualization library.Integrate Evidently at different stages of the ML pipeline – data preprocessing, model evaluation, and production monitoring – to track metrics.Log model evaluation and drift metrics to tools like MLflow and Prefect for a complete view of model health.Build custom test suites tailored to your specific data and use case by modifying its parameters.This article was published as a part of the Data Science Blogathon.Understanding ML Monitoring and Observability in AI SystemsML Monitoring and Observability are essential components of maintaining the health and performance of AI systems. Let’s delve into their significance and how they contribute to the overall effectiveness of AI models.ML MonitoringWe need ML Monitoring to do certain things: Track the behavior of the models, whose output is generated, but they are not implemented in production( Candidate models).During the comparison of 2/more candidate models (A/B tests).To track the performance of the production model.ML Monitoring is not only about the model, it’s about the overall health of the software system.It’s a combination of different layers: Service layer: where we will check the memory and overall latency taken.Data and model health layer: It is used to check data drift, data leakage, schema change, etc., We should also monitor the KPI (Key Performance Indicators) metrics of that particular business, such as customer satisfaction, financial performance, employee productivity, sales growth, and other factors.Note: Choosing the right metric to monitor the ML model, might not be the best metric all the time, continuous re-assessment is needed.ML ObservabilityML Observability is a superset of ML Monitoring. ML Monitoring refers to only finding the issues, and metrics and making the calculations, whereas observability covers the understanding of overall system behavior, specifically, finding the actual root cause for the issues that happened.Both monitoring and observability help us find the issue, and its root cause, analyze it, retrain the model, and document the quality metrics, for various team members to understand and resolve the issues.Key Considerations for ML Monitoring Create an ML Monitoring setup concerning the specific use cases.Choose model re-training concerning the use case.Choose a reference dataset for reference to compare with the batch dataset.Create Custom user-defined metrics for monitoring.Let us see about these below:ML Monitoring setup depends on the scale of complexity of deployment procedures we follow, the stability of the environment, feedback schedules, and seriousness/ impact level in case of model down, for that respective business.We can choose automated model retraining in the deployment, to make predictions. But the decision to set up an automated retraining schedule depends on a lot of factors like cost, rules, and regulations of the company, use cases, etc.,Reference Dataset in ML MonitoringSuppose in production, if we have different models and each model uses different features, which belongs to variety of structures(both structured and unstructured features), it is difficult to find the data drift and other metrics. Instead we can create a reference dataset, which has all the expected trends, it should have and also some different values, and we will compare the properties of the new batch of data with the reference dataset, to find out if there is any significant differences or not.It will serve as a baseline for distribution drift detection. Choosing the reference dataset, can be one or multiple datasets, like one for evaluating the model, other for data drift evaluation, all depends upon the use cases. We can also recreate the reference datasets based on our use cases, it may be daily/weekly/monthly using automated functions, also known as moving window strategy. So, it is important to choose a right reference dataset.Custom Metrics in ML MonitoringInstead of choosing the standard statistical metrics for evaluation like accuracy, precision, recall, and F1 score, we can create our custom metrics, that will bring more value to our specific use case. We can consider the KPIs to choose the user-defined metrics.ML Monitoring ArchitectureML Monitoring needs to collect data and performance metrics at different stages. This involves:Backend Monitoring Data pipelines: Automated scripts that analyze the model predictions, data quality, and drift, and the results are stored in a database.Batch monitoring: Scheduled jobs that run model evaluations and log metrics to a database.Real-time monitoring: Metrics are sent from live ML models to a monitoring service for tracking. Alerts: Get notifications when metric values are below thresholds without even the need for a dashboard.Reports: Static reports for one-time sharing.Dashboards: Live dashboards to interactively visualize model and data metrics over time.ML Monitoring metrics: Model Quality, Data Quality, Data DriftEvaluation of ML Model QualityTo evaluate the model quality, we should not only use the standard metrics like precision, and recall, but we should also use the custom metrics, to implement that, we should have a deep knowledge of the business. Standard ML Monitoring is not always enough, because the feedback/ ground truth is delayed, so we will use the past performance to predict, but it will not guarantee us future results, especially in a volatile environment, where our target variable changes frequently, and also different segment of categories needs different metrics, the total aggregate metrics are not enough always. To tackle this, we should do Early monitoring.Here, the below command is used to install evidently:pip install evidentlyThen, we will install all the necessary libraries.#import necessary libraries import numpy as np import pandas as pd from sklearn import ensemble from sklearn import datasets from evidently.report import Report from evidently.metric_preset import ClassificationPreset, RegressionPreset from evidently.metrics import *We will create two datasets, one is the Reference dataset, and the other one is the current dataset. Reference is the training dataset, current is the batch dataset. We will then compare these 2 datasets with Evidently to evaluate the metrics.Note: Evidently to display the metrics, needs the following features in the datasets, the ‘target’ named feature is for the target variable, ‘prediction’ named feature is only for the predicted value from the model.First, we will see a regression example. Here, we will create a simulated predicted value feature in both datasets, by adding some noise to the target feature values.# Import the necessary libraries and modules from sklearn import datasets import pandas as pd import numpy as np # Load the diabetes dataset from sklearn data = datasets.load_diabetes() # Create a DataFrame from the dataset’s features and target values diabetes = pd.DataFrame(data.data, columns=data.feature_names) diabetes[‘target’] = data.target # Add the actual target values to the DataFrame # Add a ‘prediction’ column to simulate model predictions diabetes[‘prediction’] = diabetes[‘target’].values + np.random.normal(0, 3, diabetes.shape[0]) diabetes.columns # Create reference and current datasets for comparison # These datasets are samples of the main dataset and are used for model evaluation diabetes_ref = diabetes.sample(n=50, replace=False) diabetes_cur = diabetes.sample(n=50, replace=False)Enjoy the evidently metrics:# Create a Report instance for regression with a set of predefined metrics regression_performance_report = Report(metrics=[ RegressionPreset(), # Preset is used for predefined set of regression metrics ]) # Run the report on the reference and current datasets regression_performance_report.run(reference_data=diabetes_ref.sort_index(), current_data=diabetes_cur.sort_index()) # Display the report in ‘inline’ mode regression_performance_report.show(mode=”inline”)Output:Classification Metrics:Next, we will see a classification code example with predefined metrics, and with specific metrics alone.from sklearn.ensemble import RandomForestClassifier # Load the Iris dataset data = datasets.load_iris() iris = pd.DataFrame(data.data, columns=data.feature_names) iris[‘target’] = data.target # Create a binary classification problem positive_class = 1 iris[‘target’] = (iris[‘target’] == positive_class).astype(int) # Split the dataset into reference and current data iris_ref = iris.sample(n=50, replace=False) iris_curr = iris.sample(n=50, replace=False)…
Complete Guide to Effortless ML Monitoring with Evidently.ai
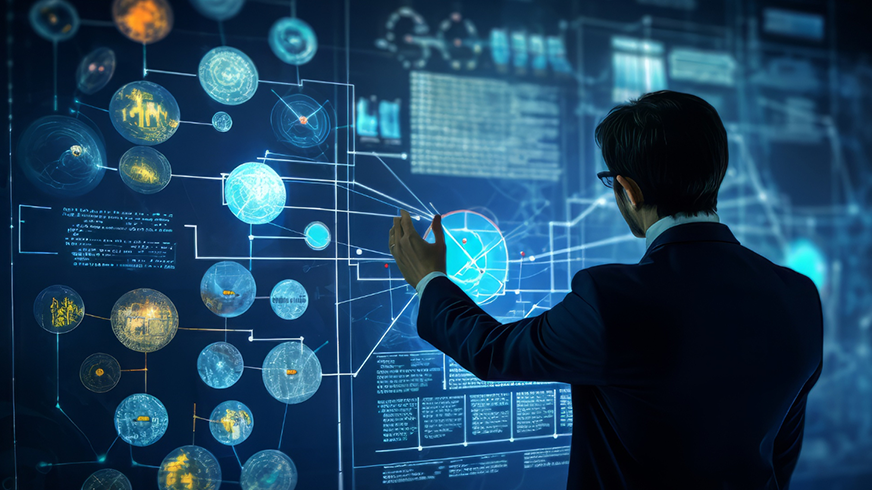
Sign Up for Our Newsletters
Get notified of the best deals on our WordPress themes.