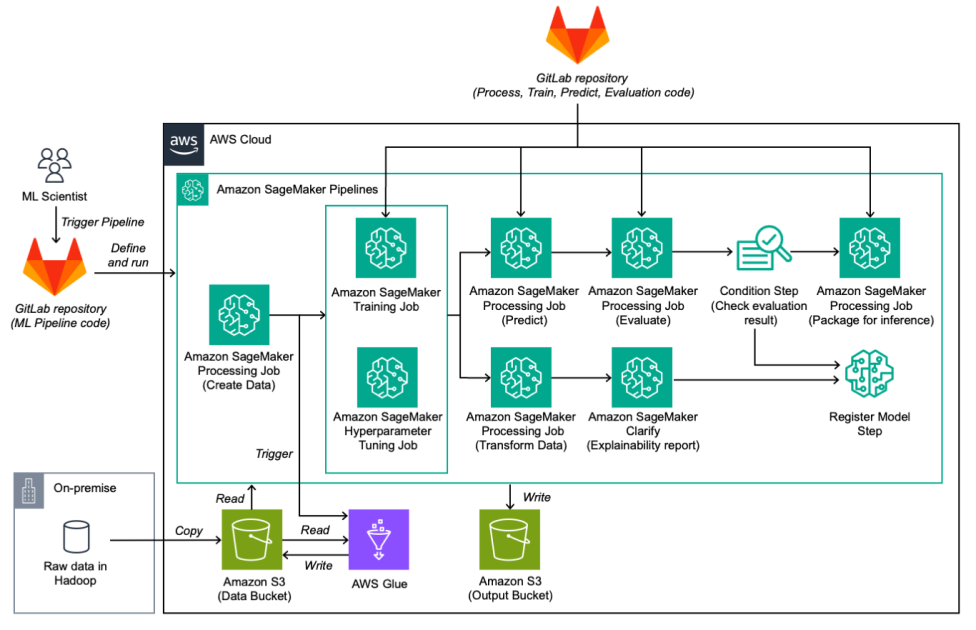
This post is co-written with Kostia Kofman and Jenny Tokar from Booking.com. As a global leader in the online travel industry, Booking.com is always seeking innovative ways to enhance its services and provide customers with tailored and seamless experiences. The Ranking team at Booking.com plays a pivotal role in ensuring that the search and recommendation algorithms are optimized to deliver the best results for their users. Sharing in-house resources with other internal teams, the Ranking team machine learning (ML) scientists often encountered long wait times to access resources for model training and experimentation – challenging their ability to rapidly experiment and innovate. Recognizing the need for a modernized ML infrastructure, the Ranking team embarked on a journey to use the power of Amazon SageMaker to build, train, and deploy ML models at scale. Booking.com collaborated with AWS Professional Services to build a solution to accelerate the time-to-market for improved ML models through the following improvements: Reduced wait times for resources for training and experimentation Integration of essential ML capabilities such as hyperparameter tuning A reduced development cycle for ML models Reduced wait times would mean that the team could quickly iterate and experiment with models, gaining insights at a much faster pace. Using SageMaker on-demand available instances allowed for a tenfold wait time reduction. Essential ML capabilities such as hyperparameter tuning and model explainability were lacking on premises. The team’s modernization journey introduced these features through Amazon SageMaker Automatic Model Tuning and Amazon SageMaker Clarify. Finally, the team’s aspiration was to receive immediate feedback on each change made in the code, reducing the feedback loop from minutes to an instant, and thereby reducing the development cycle for ML models. In this post, we delve into the journey undertaken by the Ranking team at Booking.com as they harnessed the capabilities of SageMaker to modernize their ML experimentation framework. By doing so, they not only overcame their existing challenges, but also improved their search experience, ultimately benefiting millions of travelers worldwide. Approach to modernization The Ranking team consists of several ML scientists who each need to develop and test their own model offline. When a model is deemed successful according to the offline evaluation, it can be moved to production A/B testing. If it shows online improvement, it can be deployed to all the users. The goal of this project was to create a user-friendly environment for ML scientists to easily run customizable Amazon SageMaker Model Building Pipelines to test their hypotheses without the need to code long and complicated modules. One of the several challenges faced was adapting the existing on-premises pipeline solution for use on AWS. The solution involved two key components: Modifying and extending existing code – The first part of our solution involved the modification and extension of our existing code to make it compatible with AWS infrastructure. This was crucial in ensuring a smooth transition from on-premises to cloud-based processing. Client package development – A client package was developed that acts as a wrapper around SageMaker APIs and the previously existing code. This package combines the two, enabling ML scientists to easily configure and deploy ML pipelines without coding. SageMaker pipeline configuration Customizability is key to the model building pipeline, and it was achieved through config.ini, an extensive configuration file. This file serves as the control center for all inputs and behaviors of the pipeline. Available configurations inside config.ini include: Pipeline details – The practitioner can define the pipeline’s name, specify which steps should run, determine where outputs should be stored in Amazon Simple Storage Service (Amazon S3), and select which datasets to use AWS account details – You can decide which Region the pipeline should run in and which role should be used Step-specific configuration – For each step in the pipeline, you can specify details such as the number and type of instances to use, along with relevant parameters The following code shows an example configuration file: [BUILD] pipeline_name = ranking-pipeline steps = DATA_TRANFORM, TRAIN, PREDICT, EVALUATE, EXPLAIN, REGISTER, UPLOAD train_data_s3_path = s3://… … [AWS_ACCOUNT] region = eu-central-1 … [DATA_TRANSFORM_PARAMS] input_data_s3_path = s3://… compression_type = GZIP …. [TRAIN_PARAMS] instance_count = 3 instance_type = ml.g5.4xlarge epochs = 1 enable_sagemaker_debugger = True … [PREDICT_PARAMS] instance_count = 3 instance_type = ml.g5.4xlarge … [EVALUATE_PARAMS] instance_type = ml.m5.8xlarge batch_size = 2048 … [EXPLAIN_PARAMS] check_job_instance_type = ml.c5.xlarge generate_baseline_with_clarify = False …. config.ini is a version-controlled file managed by Git, representing the minimal configuration required for a successful training pipeline run. During development, local configuration files that are not version-controlled can be utilized. These local configuration files only need to contain settings relevant to a specific run, introducing flexibility without complexity. The pipeline creation client is designed to handle multiple configuration files, with the latest one taking precedence over previous settings. SageMaker pipeline steps The pipeline is divided into the following steps: Train and test data preparation – Terabytes of raw data are copied to an S3 bucket, processed using AWS Glue jobs for Spark processing, resulting in data structured and formatted for compatibility. Train – The training step uses the TensorFlow estimator for SageMaker training jobs. Training occurs in a distributed manner using Horovod, and the resulting model artifact is stored in Amazon S3. For hyperparameter tuning, a hyperparameter optimization (HPO) job can be initiated, selecting the best model based on the objective metric. Predict – In this step, a SageMaker Processing job uses the stored model artifact to make predictions. This process runs in parallel on available machines, and the prediction results are stored in Amazon S3. Evaluate – A PySpark processing job evaluates the model using a custom Spark script. The evaluation report is then stored in Amazon S3. Condition – After evaluation, a decision is made regarding the model’s quality. This decision is based on a condition metric defined in the configuration file. If the evaluation is positive, the model is registered as approved; otherwise, it’s registered as rejected. In both cases, the evaluation and explainability report, if generated, are recorded in the model registry. Package model for inference – Using a processing job, if the evaluation results are positive, the model is packaged, stored in Amazon S3, and made ready for upload to the internal ML portal. Explain – SageMaker Clarify generates an explainability report. Two distinct repositories are used. The first repository contains the definition and build code for the ML pipeline, and the second repository contains the code that runs inside each step, such as processing, training, prediction, and evaluation. This dual-repository approach allows for greater modularity, and enables science and engineering teams to iterate independently on ML code and ML pipeline components. The following diagram illustrates the solution workflow. Automatic model tuning Training ML models requires an iterative approach of multiple training experiments to build a robust and performant final model for business use. The ML scientists have to select the appropriate model type, build the correct input datasets, and adjust the set of hyperparameters that control the model learning process during training. The selection of appropriate values for hyperparameters for the model training process can significantly influence the final performance of the model. However, there is no unique or defined way to determine which values are appropriate for a specific use case. Most of the time, ML scientists will need to run multiple training jobs with slightly different sets of hyperparameters, observe the model training metrics, and then try to select more promising values for the next iteration. This process of tuning model performance is also known as hyperparameter optimization (HPO), and can at times require hundreds of experiments. The Ranking team used to perform HPO manually in their on-premises environment because they could only launch a very limited number of training jobs in parallel. Therefore, they had to run HPO sequentially, test and select different combinations of hyperparameter values manually, and regularly monitor progress. This prolonged the model development and tuning process and limited the overall number of HPO experiments that could run in a feasible amount of time. With the move to AWS, the Ranking team was able to use the automatic model tuning (AMT) feature of SageMaker. AMT enables Ranking ML scientists to automatically launch hundreds of training jobs within hyperparameter ranges of interest to find the best performing version of the final model according to the chosen metric. The Ranking team is now able choose between four different automatic tuning strategies for their hyperparameter selection: Grid search – AMT…