At AWS re:Invent 2023, we announced the general availability of Knowledge Bases for Amazon Bedrock. With a knowledge base, you can securely connect foundation models (FMs) in Amazon Bedrock to your company data for fully managed Retrieval Augmented Generation (RAG). In a previous post, we described how Knowledge Bases for Amazon Bedrock manages the end-to-end RAG workflow for you and shared details about some of the recent feature launches. For RAG-based applications, the accuracy of the generated response from large language models (LLMs) is dependent on the context provided to the model. Context is retrieved from the vector database based on the user query. Semantic search is widely used because it is able to understand more human-like questions—a user’s query is not always directly related to the exact keywords in the content that answers it. Semantic search helps provide answers based on the meaning of the text. However, it has limitations in capturing all the relevant keywords. Its performance relies on the quality of the word embeddings used to represent meaning of the text. To overcome such limitations, combining semantic search with keyword search (hybrid) will give better results. In this post, we discuss the new feature of hybrid search, which you can select as a query option alongside semantic search. Hybrid search overview Hybrid search takes advantage of the strengths of multiple search algorithms, integrating their unique capabilities to enhance the relevance of returned search results. For RAG-based applications, semantic search capabilities are commonly combined with traditional keyword-based search to improve the relevance of search results. It enables searching over both the content of documents and their underlying meaning. For example, consider the following query: What is the cost of the book “<book_name>” on <website_name>? In this query for a book name and website name, a keyword search will give better results, because we want the cost of the specific book. However, the term “cost” might have synonyms such as “price,” so it will be better to use semantic search, which understands the meaning of the text. Hybrid search brings the best of both approaches: precision of semantic search and coverage of keywords. It works great for RAG-based applications where the retriever has to handle a wide variety of natural language queries. The keywords help cover specific entities in the query such as product name, color, and price, while semantics better understands the meaning and intent within the query. For example, if you have want to build a chatbot for an ecommerce website to handle customer queries such as the return policy or details of the product, using hybrid search will be most suitable. Use cases for hybrid search The following are some common use cases for hybrid search: Open domain question answering – This involves answering questions on a wide variety of topics. This requires searching over large collections of documents with diverse content, such as website data, which can include various topics such as sustainability, leadership, financial results, and more. Semantic search alone can’t generalize well for this task, because it lacks the capacity for lexical matching of unseen entities, which is important for handling out-of-domain examples. Therefore, combining keyword-based search with semantic search can help narrow down the scope and provide better results for open domain question answering. Contextual-based chatbots – Conversations can rapidly change direction and cover unpredictable topics. Hybrid search can better handle such open-ended dialogs. Personalized search – Web-scale search over heterogeneous content benefits from a hybrid approach. Semantic search handles popular head queries, while keywords cover rare long-tail queries. Although hybrid search offers wider coverage by combining two approaches, semantic search has precision advantages when the domain is narrow and semantics are well-defined, or when there is little room for misinterpretation, like factoid question answering systems. Benefits of hybrid search Both keyword and semantic search will return a separate set of results along with their relevancy scores, which are then combined to return the most relevant results. Knowledge Bases for Amazon Bedrock currently supports four vector stores: Amazon OpenSearch Serverless, Amazon Aurora PostgreSQL-Compatible Edition, Pinecone, and Redis Enterprise Cloud. As of this writing, the hybrid search feature is available for OpenSearch Serverless, with support for other vector stores coming soon. The following are some of the benefits of using hybrid search: Improved accuracy – The accuracy of the generated response from the FM is directly dependent on the relevancy of retrieved results. Based on your data, it can be challenging to improve the accuracy of your application only using semantic search. The key benefit of using hybrid search is to get improved quality of retrieved results, which in turn helps the FM generate more accurate answers. Expanded search capabilities – Keyword search casts a wider net and finds documents that may be relevant but might not contain semantic structure throughout the document. It allows you to search on keywords as well as the semantic meaning of the text, thereby expanding the search capabilities. In the following sections, we demonstrate how to use hybrid search with Knowledge Bases for Amazon Bedrock. Use hybrid search and semantic search options via SDK When you call the Retrieve API, Knowledge Bases for Amazon Bedrock selects the right search strategy for you to give you most relevant results. You have the option to override it to use either hybrid or semantic search in the API. Retrieve API The Retrieve API is designed to fetch relevant search results by providing the user query, knowledge base ID, and number of results that you want the API to return. This API converts user queries into embeddings, searches the knowledge base using either hybrid search or semantic (vector) search, and returns the relevant results, giving you more control to build custom workflows on top of the search results. For example, you can add postprocessing logic to the retrieved results or add your own prompt and connect with any FM provided by Amazon Bedrock for generating answers. To show you an example of switching between hybrid and semantic (vector) search options, we have created a knowledge base using the Amazon 10K document for 2023. For more details on creating a knowledge base, refer to Build a contextual chatbot application using Knowledge Bases for Amazon Bedrock. To demonstrate the value of hybrid search, we use the following query: As of December 31st 2023, what is the leased square footage for physical stores in North America? The answer for the preceding query involves a few keywords, such as the date, physical stores, and North America. The correct response is 22,871 thousand square feet. Let’s observe the difference in the search results for both hybrid and semantic search. The following code shows how to use hybrid or semantic (vector) search using the Retrieve API with Boto3: import boto3 bedrock_agent_runtime = boto3.client( service_name = “bedrock-agent-runtime” ) def retrieve(query, kbId, numberOfResults=5): return bedrock_agent_runtime.retrieve( retrievalQuery= { ‘text’: query }, knowledgeBaseId=kbId, retrievalConfiguration= { ‘vectorSearchConfiguration’: { ‘numberOfResults’: numberOfResults, ‘overrideSearchType’: “HYBRID/SEMANTIC”, # optional } } ) response = retrieve(“As of December 31st 2023, what is the leased square footage for physical stores in North America?”, “<knowledge base id>”)[“retrievalResults”] The overrideSearchType option in retrievalConfiguration offers the choice to use either HYBRID or SEMANTIC. By default, it will select the right strategy for you to give you most relevant results, and if you want to override the default option to use either hybrid or semantic search, you can set the value to HYBRID/SEMANTIC. The output of the Retrieve API includes the retrieved text chunks, the location type and URI of the source data, and the relevancy scores of the retrievals. The scores help determine which chunks best match the response of the query. The following are the results for the preceding query using hybrid search (with some of the output redacted for brevity): [ { “content”: { “text”: “… Description of Use Leased Square Footage (1)…. Physical stores (2) 22,871 …” }, “location”: { “type”: “S3”, “s3Location”: { “uri”: “s3://<bucket_name>/amazon-10k-2023.pdf” } }, “score”: 0.6389407 }, { “content”: { “text”: “Property and equipment, net by segment is as follows (in millions): December 31, 2021 2022 2023 North America $ 83,640 $ 90,076 $ 93,632 International 21,718 23,347 24,357 AWS 43,245 60,324 72,701 Corporate 1..” }, “location”: { “type”: “S3”, “s3Location”: { “uri”: “s3://<bucket_name>/amazon-10k-2023.pdf” } }, “score”: 0.6389407 }, { “content”: { “text”: “..amortization of property and equipment acquired under finance leases of $9.9 billion, $6.1 billion, and $5.9 billion for 2021, 2022, and 2023.…
Knowledge Bases for Amazon Bedrock now supports hybrid search
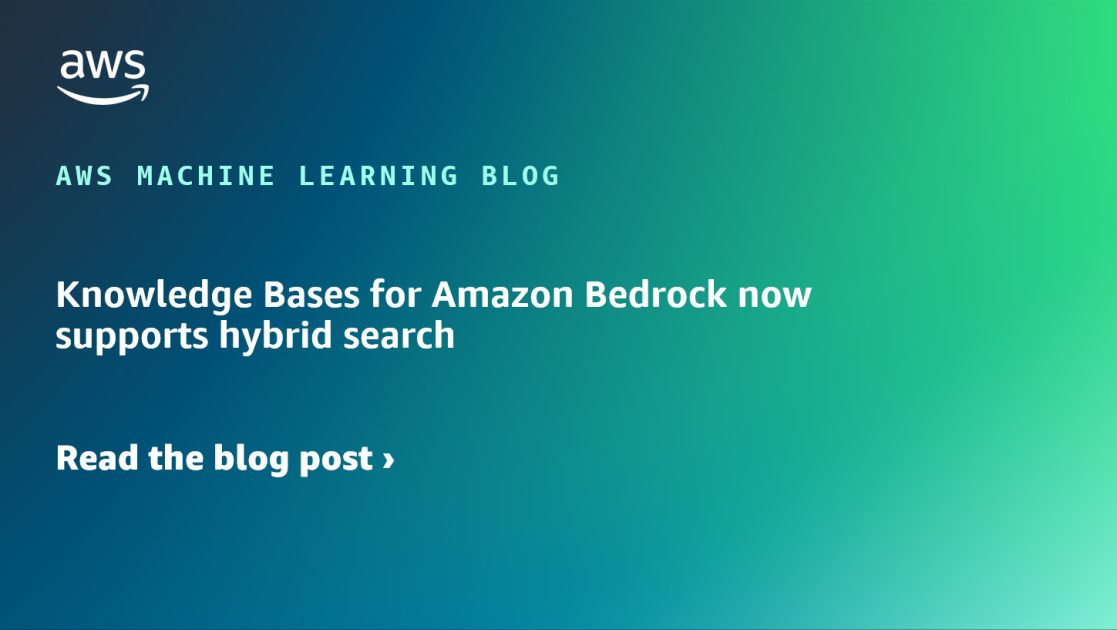
Sign Up for Our Newsletters
Get notified of the best deals on our WordPress themes.