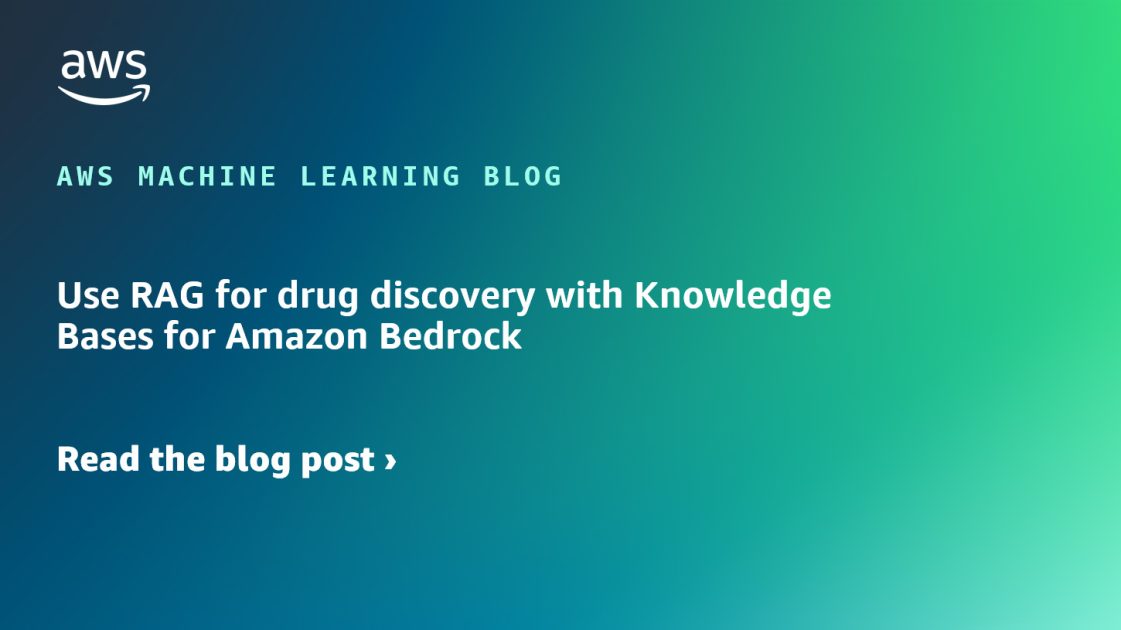
Amazon Bedrock provides a broad range of models from Amazon and third-party providers, including Anthropic, AI21, Meta, Cohere, and Stability AI, and covers a wide range of use cases, including text and image generation, embedding, chat, high-level agents with reasoning and orchestration, and more. Knowledge Bases for Amazon Bedrock allows you to build performant and customized Retrieval Augmented Generation (RAG) applications on top of AWS and third-party vector stores using both AWS and third-party models. Knowledge Bases for Amazon Bedrock automates synchronization of your data with your vector store, including diffing the data when it’s updated, document loading, and chunking, as well as semantic embedding. It allows you to seamlessly customize your RAG prompts and retrieval strategies—we provide the source attribution, and we handle memory management automatically. Knowledge Bases is completely serverless, so you don’t need to manage any infrastructure, and when using Knowledge Bases, you’re only charged for the models, vector databases and storage you use. RAG is a popular technique that combines the use of private data with large language models (LLMs). RAG starts with an initial step to retrieve relevant documents from a data store (most commonly a vector index) based on the user’s query. It then employs a language model to generate a response by considering both the retrieved documents and the original query. In this post, we demonstrate how to build a RAG workflow using Knowledge Bases for Amazon Bedrock for a drug discovery use case. Overview of Knowledge Bases for Amazon Bedrock Knowledge Bases for Amazon Bedrock supports a broad range of common file types, including .txt, .docx, .pdf, .csv, and more. To enable effective retrieval from private data, a common practice is to first split these documents into manageable chunks. Knowledge Bases has implemented a default chunking strategy that works well in most cases to allow you to get started faster. If you want more control, Knowledge Bases lets you control the chunking strategy through a set of preconfigured options. You can control the maximum token size and the amount of overlap to be created across chunks to provide coherent context to the embedding. Knowledge Bases for Amazon Bedrock manages the process of synchronizing data from your Amazon Simple Storage Service (Amazon S3) bucket, splits it into smaller chunks, generates vector embeddings, and stores the embeddings in a vector index. This process comes with intelligent diffing, throughput, and failure management. At runtime, an embedding model is used to convert the user’s query to a vector. The vector index is then queried to find documents similar to the user’s query by comparing document vectors to the user query vector. In the final step, semantically similar documents retrieved from the vector index are added as context for the original user query. When generating a response for the user, the semantically similar documents are prompted in the text model, together with source attribution for traceability. Knowledge Bases for Amazon Bedrock supports multiple vector databases, including Amazon OpenSearch Serverless, Amazon Aurora, Pinecone, and Redis Enterprise Cloud. The Retrieve and RetrieveAndGenerate APIs allow your applications to directly query the index using a unified and standard syntax without having to learn separate APIs for each different vector database, reducing the need to write custom index queries against your vector store. The Retrieve API takes the incoming query, converts it into an embedding vector, and queries the backend store using the algorithms configured at the vector database level; the RetrieveAndGenerate API uses a user-configured LLM provided by Amazon Bedrock and generates the final answer in natural language. The native traceability support informs the requesting application about the sources used to answer a question. For enterprise implementations, Knowledge Bases supports AWS Key Management Service (AWS KMS) encryption, AWS CloudTrail integration, and more. In the following sections, we demonstrate how to build a RAG workflow using Knowledge Bases for Amazon Bedrock, backed by the OpenSearch Serverless vector engine, to analyze an unstructured clinical trial dataset for a drug discovery use case. This data is information rich but can be vastly heterogenous. Proper handling of specialized terminology and concepts in different formats is essential to detect insights and ensure analytical integrity. With Knowledge Bases for Amazon Bedrock, you can access detailed information through simple, natural queries. Build a knowledge base for Amazon Bedrock In this section, we demo the process of creating a knowledge base for Amazon Bedrock via the console. Complete the following steps: On the Amazon Bedrock console, under Orchestration in the navigation pane, choose Knowledge base. Choose Create knowledge base. In the Knowledge base details section, enter a name and optional description. In the IAM permissions section, select Create and use a new service role. For Service name role, enter a name for your role, which must start with AmazonBedrockExecutionRoleForKnowledgeBase_. Choose Next. In the Data source section, enter a name for your data source and the S3 URI where the dataset sits. Knowledge Bases supports the following file formats: Plain text (.txt) Markdown (.md) HyperText Markup Language (.html) Microsoft Word document (.doc/.docx) Comma-separated values (.csv) Microsoft Excel spreadsheet (.xls/.xlsx) Portable Document Format (.pdf) Under Additional settings¸ choose your preferred chunking strategy (for this post, we choose Fixed size chunking) and specify the chunk size and overlay in percentage. Alternatively, you can use the default settings. Choose Next. In the Embeddings model section, choose the Titan Embeddings model from Amazon Bedrock. In the Vector database section, select Quick create a new vector store, which manages the process of setting up a vector store. Choose Next. Review the settings and choose Create knowledge base. Wait for the knowledge base creation to complete and confirm its status is Ready. In the Data source section, or on the banner at the top of the page or the popup in the test window, choose Sync to trigger the process of loading data from the S3 bucket, splitting it into chunks of the size you specified, generating vector embeddings using the selected text embedding model, and storing them in the vector store managed by Knowledge Bases for Amazon Bedrock. The sync function supports ingesting, updating, and deleting the documents from the vector index based on changes to documents in Amazon S3. You can also use the StartIngestionJob API to trigger the sync via the AWS SDK. When the sync is complete, the Sync history shows status Completed. Query the knowledge base In this section, we demonstrate how to access detailed information in the knowledge base through straightforward and natural queries. We use an unstructured synthetic dataset consisting of PDF files, the page number of each ranging from 10–100 pages, simulating a clinical trial plan of a proposed new medicine including statistical analysis methods and participant consent forms. We use the Knowledge Bases for Amazon Bedrock retrieve_and_generate and retrieve APIs with Amazon Bedrock LangChain integration. Before you can write scripts that use the Amazon Bedrock API, you’ll need to install the appropriate version of the AWS SDK in your environment. For Python scripts, this will be the AWS SDK for Python (Boto3): pip install langchain pip install boto3 Additionally, enable access to the Amazon Titan Embeddings model and Anthropic Claude v2 or v1. For more information, refer to Model access. Generate questions using Amazon Bedrock We can use Anthropic Claude 2.1 for Amazon Bedrock to propose a list of questions to ask on the clinical trial dataset: import boto3 from langchain.llms.bedrock import Bedrock bedrock_client = boto3.client(“bedrock-runtime”) # Start with the query prompt = “For medical research trial consent forms to sign, what are the top 5 questions can be asked?” claude_llm = Bedrock( model_id=”anthropic.claude-v2:1″, model_kwargs={“temperature”: 0, “top_k”: 10, “max_tokens_to_sample”: 3000}, client=bedrock_client, ) # Provide the prompt to the LLM to generate an answer to the query without any additional context provided response = claude_llm(prompt) questions = [ item.split(“.”)[1].strip() for item in response.strip().split(“\n\n”)[1:-1] ] questions >>> answer: ‘What is the purpose of the study? Make sure you understand the goals of the research and what the study procedures will entail’, ‘What are the risks and potential benefits? The form should explain all foreseeable risks, side effects, or discomforts you might experience from participating’, ‘What will participation involve? Get details on what tests, medications, lifestyle changes, or procedures you will go through, how much time it will take, and how long the study will last’, ‘Are there any costs or payments? Ask if you will be responsible for any costs related to the study or get paid for participating’,…